In today’s job market, having an impressive LinkedIn profile is just as important as having a great resume. Especially for first-time job-seekers, college students and fresh graduates, LinkedIn can be…
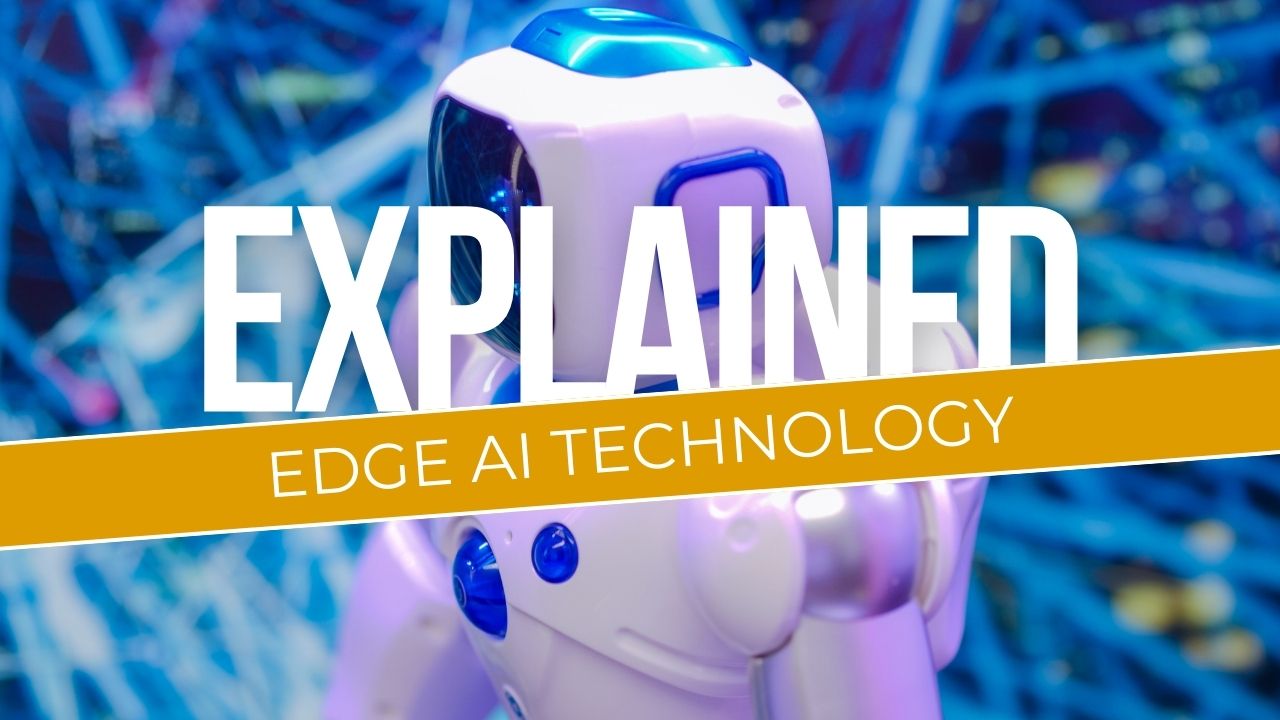
What is Edge AI and How Does It Work?
With the rapid growth of smart devices and real-time applications, Edge AI has emerged as one of the most exciting developments in technology today. From self-driving cars and wearable health devices to smart cities and industrial automation, Edge AI is making computing more responsive, secure, and efficient.
If you’re a college student or fresher in India interested in the future of AI, machine learning, or embedded systems, this comprehensive guide is for you. In this article, you’ll learn what Edge AI is, how it works, how it’s different from cloud AI, and where it’s heading.
What is Edge AI?
Edge Artificial Intelligence, commonly known as Edge AI, refers to AI algorithms that process data directly on a local device, at the ‘edge’ of the network, rather than sending that data to a remote server or cloud. This means the AI processing happens right where the data is generated – on your smartphone, IoT sensors, surveillance cameras, industrial equipment, or any other edge device.
In traditional AI implementations, devices collect data, send it to the cloud for processing, and then receive back the results. Edge AI eliminates this round trip, enabling devices to make decisions locally and autonomously. This is particularly valuable in scenarios where connectivity is limited, bandwidth is expensive, or when real-time response is critical.
How Edge AI Works
Edge AI functions through a process of model optimization and deployment that brings the intelligence directly to the device. Here’s how it works:
Model Development and Optimization
The process begins with developing an AI model using traditional methods, training on powerful servers or cloud infrastructure using large datasets. However, these original models are typically too large and computationally demanding to run on edge devices with limited resources.
Therefore, engineers use various optimization techniques:
- Model Compression: Reducing the size of neural networks through pruning (removing less important connections), quantization (using fewer bits to represent weights), and knowledge distillation (creating smaller models that mimic larger ones).
- Hardware-Specific Optimization: Tailoring models to run efficiently on specific edge hardware, such as mobile GPUs, neural processing units (NPUs), or specialized AI accelerators.
- Algorithm Selection: Choosing or designing algorithms that balance accuracy with computational efficiency, often trading some precision for dramatic improvements in speed and energy consumption.
Edge Deployment Architecture
Once optimized, these leaner models are deployed to edge devices, where they operate within specific architectural constraints:
- On-Device Processing: The AI models run directly on the device’s hardware, using local computational resources.
- Hybrid Approaches: Some systems use a combination of edge and cloud processing. Simple, time-sensitive tasks happen on the device, while more complex analysis occurs in the cloud.
- Distributed Intelligence: In more advanced setups, AI processing may be distributed across multiple edge devices in a mesh network, allowing for collaborative intelligence.
Inference Process
When an edge device encounters new data (like a camera seeing an image or a microphone hearing a sound), it runs this data through its local AI model in a process called inference. The device can then make immediate decisions based on the AI’s analysis without waiting for external input.
For example, a security camera with Edge AI can instantly recognize authorized personnel without sending footage to a central server, or a smart agricultural sensor can immediately adjust irrigation systems based on soil conditions.
Benefits of Edge AI
Edge AI offers several compelling advantages that make it particularly relevant for India’s unique technology landscape and challenges:
- Reduced Latency: By processing data locally, Edge AI eliminates network delays. This near-instant response time is critical for applications like autonomous vehicles, industrial automation, and real-time monitoring systems where milliseconds matter. In India, where network connectivity can still be challenging in many regions, this local processing capability ensures consistent performance regardless of internet availability.
- Improved Privacy and Security: With Edge AI, sensitive data never leaves the device, providing inherent privacy benefits. This is increasingly important as data protection regulations become more stringent globally and in India, where the Digital Personal Data Protection Act places new emphasis on data sovereignty. Healthcare applications, for instance, can analyze sensitive patient data locally without transmitting it across networks, reducing both compliance concerns and security risks.
- Bandwidth and Cost Efficiency: By processing data locally and only sending relevant insights to the cloud, Edge AI dramatically reduces the amount of data transmitted over networks. This is particularly valuable in India, where data costs can still represent a significant expense for users and businesses alike. For example, a smart surveillance system using Edge AI might only upload video clips when it detects unusual activity, rather than streaming all footage continuously.
- Operational Reliability: Edge AI systems can function even when network connectivity is unavailable or unreliable. This independence from constant connectivity makes Edge AI solutions particularly well-suited for deployment in India’s rural areas or locations with infrastructure challenges.
- Energy Efficiency: Optimized Edge AI models typically consume less power than constantly transmitting data to remote servers for processing. This energy efficiency extends battery life in mobile devices and reduces operational costs in industrial applications. For a country like India that is focused on sustainable development and energy conservation, this benefit aligns perfectly with broader national goals.
Edge AI vs. Cloud AI
Understanding the differences between Edge AI and Cloud AI helps clarify when each approach is most appropriate:
Feature | Edge AI | Cloud AI |
Location of Processing | On-device (local) | Remote data centers |
Latency | Very low | Higher |
Internet Dependency | Minimal | High |
Security | Better local control | Risk of breaches in transit |
Power Consumption | Optimized for low-power devices | High computational resources |
Examples | Smart cameras, drones, and smartwatches | Chatbots, recommendation engines, virtual assistants |
In practice, many systems use a hybrid approach. For example, a smart manufacturing system in an Indian factory might use Edge AI for immediate quality control decisions on the production line, while sending aggregated data to the cloud for long-term analytics and process optimization.
The choice between Edge AI and Cloud AI isn’t binary, it’s about finding the right balance for specific use cases, considering factors like connectivity, privacy requirements, latency needs, and computational demands.
Examples of Edge AI Use Cases
Edge AI is already transforming numerous industries in India and worldwide. Here are some compelling applications that demonstrate its versatility and impact:
Healthcare and Telemedicine
In rural India, where access to specialists may be limited, Edge AI-powered diagnostic tools are making a significant difference. Devices like portable ultrasound machines with built-in AI can help frontline health workers identify potential complications during pregnancy without requiring constant internet connectivity.
Similarly, Edge AI-enabled stethoscopes can analyze heart and lung sounds in real-time, flagging potential issues that might otherwise go undetected until a patient sees a specialist.
Agriculture and Rural Development
India’s agricultural sector is being revolutionized by Edge AI. Smart sensors deployed across farms can monitor soil moisture, nutrient levels, and plant health in real-time, making autonomous decisions about irrigation and fertilization.
These systems operate independently of network connectivity, providing farmers with actionable insights regardless of their location’s infrastructure. For example, companies like Fasal and CropIn are deploying Edge AI solutions that have helped increase yields by 20-30% while reducing water usage.
Manufacturing and Industry 4.0
Indian manufacturers are increasingly adopting Edge AI for quality control and predictive maintenance. AI-enabled cameras can inspect products on assembly lines at high speeds, identifying defects that would be invisible to the human eye.
Meanwhile, vibration sensors with embedded AI can monitor machinery health, detecting subtle changes that might indicate impending failures long before they occur. These systems can prevent costly downtime without requiring continuous cloud connectivity on the factory floor.
Smart Cities and Urban Infrastructure
As India continues its Smart Cities Mission, Edge AI is playing a crucial role in traffic management, public safety, and resource optimization. AI-enabled traffic cameras can adjust signal timing based on current conditions, reducing congestion without sending constant video streams to central servers.
Similarly, Edge AI-powered environmental sensors can monitor air quality and noise pollution, creating hyperlocal data maps that inform urban planning decisions.
Consumer Electronics and Mobile Computing
Perhaps the most visible implementation of Edge AI for many Indians is in their smartphones. Features like voice assistants, camera enhancements, and predictive text all leverage on-device AI to deliver personalized experiences while preserving privacy.
For example, the computational photography capabilities in modern smartphones use Edge AI to enhance low-light photos, identify subjects, and apply appropriate effects—all happening locally on the device in a fraction of a second.
What Role Does Cloud Computing Play in Edge Computing?
While Edge AI focuses on local processing, cloud computing remains an essential part of the overall AI ecosystem. The relationship between edge and cloud is complementary rather than competitive, with each handling different aspects of the AI workflow:
Model Development and Training
The cloud provides the vast computational resources needed to train sophisticated AI models using large datasets. These resources would be impossible to replicate on edge devices. For example, training a computer vision model might require processing millions of images—a task well-suited to cloud infrastructure.
Continuous Learning and Improvement
Edge devices can send anonymized insights back to the cloud, where this collective data improves central models. These enhanced models are then periodically deployed back to edge devices, creating a virtuous cycle of improvement.
This approach, sometimes called “federated learning,” allows devices to contribute to model improvement without sharing raw data, addressing both privacy concerns and bandwidth limitations.
Complex Analysis and Long-term Storage
While edge devices handle immediate processing needs, the cloud remains ideal for deeper analysis that isn’t time-sensitive. For instance, a manufacturing plant might use Edge AI for real-time quality control but send aggregated production statistics to the cloud for trend analysis and process optimization.
Orchestration and Management
Cloud platforms provide the infrastructure to manage fleets of edge devices, handling tasks like:
- Deployment of updated AI models
- Monitoring device health and performance
- Security patches and updates
- Configuration management
- Analytics across distributed systems
Edge-Cloud Continuum
Rather than viewing edge and cloud as separate domains, modern systems often operate along a continuum, with processing happening at the most appropriate point based on factors like latency requirements, available bandwidth, and computational needs.
For Indian technology professionals, understanding how to architect solutions across this continuum represents a valuable skill set as organizations increasingly adopt hybrid approaches.
Future of Edge AI
As we look toward the horizon, several trends are shaping the evolution of Edge AI, creating exciting opportunities for India’s tech workforce:
Increasingly Powerful Edge Hardware
The development of specialized AI accelerators and more efficient processors is dramatically expanding what’s possible at the edge. Companies like MediaTek, Qualcomm, and others are creating increasingly powerful AI hardware specifically designed for edge devices.
These advancements will enable more sophisticated applications to run locally, from real-time language translation to advanced computer vision capabilities on everyday devices.
5G Integration
The rollout of 5G networks across India will create new possibilities for Edge AI by providing high-bandwidth, low-latency connectivity between edge devices and cloud resources when needed. This will enable more flexible hybrid architectures where processing can dynamically shift between edge and cloud based on current conditions.
Tiny ML and Ultra-Efficient Models
The emerging field of TinyML focuses on developing machine learning models that can run on microcontrollers and extremely resource-constrained devices. This approach will expand Edge AI to entirely new categories of devices, from wearable health monitors to smart agricultural sensors that can operate for years on a single battery.
Collaborative and Federated Learning
Future Edge AI systems will increasingly collaborate, sharing insights while preserving data privacy. Federated learning approaches allow devices to collectively improve models without centralizing sensitive data, addressing both privacy concerns and bandwidth limitations.
This approach is particularly relevant for India, where data protection regulations are evolving and where connectivity challenges can make the traditional centralized model training impractical.
Environmental Impact
As sustainability becomes an increasing focus globally, Edge AI’s energy efficiency advantages will become more prominent. By processing data locally and reducing transmission needs, Edge AI can significantly lower the carbon footprint of AI implementations compared to cloud-only approaches.
Job and Skill Implications
For Indian college students and recent graduates, the rise of Edge AI creates demand for specific technical skills:
- Embedded systems programming
- Model optimization and compression techniques
- Hardware-software co-design
- Distributed systems architecture
- Security for edge devices
Leading technology companies, startups, and research institutions in India are already investing heavily in Edge AI capabilities, creating promising career paths in this emerging field.
Edge AI is not just a tech buzzword, it’s the future of intelligent computing. It enables real-time decision-making, enhances data privacy, and opens up a new world of innovation, especially for emerging markets like India.
Whether you’re a computer science student or an electronics engineering fresher, now is the right time to dive into what Edge AI is, learn how Edge AI works, and explore real-world applications.
FAQs on Edge AI
What are the hardware requirements for implementing Edge AI solutions?
Edge AI requires specialized hardware like Neural Processing Units (NPUs), Field-Programmable Gate Arrays (FPGAs), or edge-optimized GPUs that balance computational power with energy efficiency while supporting neural network operations in resource-constrained environments.
How does model compression work in Edge AI development?
Model compression in Edge AI reduces neural network size through techniques like quantization (using fewer bits for weights), pruning (removing unnecessary connections), and knowledge distillation (transferring learning from large models to smaller ones), making models deployable on resource-limited devices.
What programming languages are best for Edge AI development?
Python remains dominant for model development, but C/C++ is crucial for optimized edge deployment. TensorFlow Lite, PyTorch Mobile, and ONNX Runtime are popular frameworks, while specialized languages like Rust are gaining traction for memory-safe edge implementations.
How does Edge AI improve data privacy compared to cloud AI?
Edge AI processes sensitive data locally on devices without transmitting it to external servers, significantly reducing data breach risks, helping companies comply with regulations like GDPR and India’s Data Protection Act, and building stronger user trust through privacy-by-design architecture.
What are the limitations of Edge AI compared to cloud-based solutions?
Edge AI faces constraints including limited computational resources restricting model complexity, challenges in model updates across distributed devices, potential inconsistencies between devices, higher hardware costs, and development complexity requiring specialized optimization skills.
How is Edge AI transforming healthcare applications?
Edge AI enables real-time patient monitoring with privacy protection, powers diagnostic tools that function in connectivity-challenged rural areas, enhances medical imaging analysis at point-of-care, and enables wearable devices that provide continuous health insights without constant cloud connectivity.
What role does Edge AI play in autonomous vehicles?
Edge AI enables autonomous vehicles to make split-second driving decisions by processing sensor data locally, recognizing objects, detecting lane positions, and identifying traffic signals with sub-millisecond latency, which is critical for passenger safety in unpredictable traffic environments.
How can TinyML accelerate Edge AI adoption?
TinyML brings machine learning capabilities to ultra-low-power microcontrollers consuming milliwatts of power, enabling AI in previously impractical applications like environmental sensors, wearables, and agricultural monitoring systems that can operate for years on a single battery charge.
What security challenges exist in Edge AI implementation?
Edge AI security challenges include physical device vulnerabilities, protection against model extraction attacks, securing the update pipeline, preventing adversarial examples that mislead models, and implementing robust authentication while maintaining performance on resource-constrained hardware.
How does federated learning enhance Edge AI capabilities?
Federated learning allows Edge AI devices to collectively improve models by sharing insights rather than raw data, enabling privacy-preserving distributed learning where devices train on local data and only exchange model updates, addressing both bandwidth limitations and data privacy concerns.
What is the impact of Edge AI on IoT scalability?
Edge AI dramatically improves IoT scalability by reducing bandwidth requirements through local data processing, enabling autonomous operation without cloud dependence, extending battery life through efficient computation, and allowing effective deployment in connectivity-challenged environments.
How can businesses calculate ROI for Edge AI implementation?
Businesses should calculate Edge AI ROI by assessing bandwidth savings, latency improvements, privacy compliance benefits, operational reliability gains, and device lifespan extension, while accounting for hardware costs, specialized development expertise, and maintenance requirements across distributed systems.
Latest Posts
Data Science vs Data Analytics – Everything You Need to Know!
In today’s digital economy, Data Science and Data Analytics have become some of the most sought-after career paths for students and freshers, especially in India. With businesses increasingly relying on…
Large Language Models Explained: How LLMs Are Transforming Technology
In today’s AI-driven world, Large Language Models (LLMs) are transforming how we interact with technology. From chatbots and virtual assistants to search engines and translation tools, LLMs are becoming an…
How to Start an AI Career in India: Jobs, Skills, Preparation Guide
Artificial Intelligence (AI) is revolutionizing industries across the globe. From automating routine tasks to enabling self-driving cars and intelligent healthcare diagnostics, AI is reshaping the future of work. For college…
Artificial Intelligence in 2025: Applications, Ethics, and Impact
In today’s rapidly evolving technological landscape, artificial intelligence (AI) stands at the forefront of innovation, reshaping industries and transforming the way we live and work. As college students and recent…
Popular Posts
Top 21 Highest Paying Jobs in India For Freshers
The Indian job market is evolving rapidly, with new opportunities emerging across various sectors. As a student or fresher, identifying the best career in India that aligns with your interests…
Best CV Formats for Freshers: Simple, Professional & Job-Winning Templates
Creating an effective CV (Curriculum Vitae) is the first step towards landing your dream job or internship as a fresh graduate. Your CV is your initial introduction to potential employers…
25+ Best Online Courses for Graduates in 2025 [Free & Certified]
In today’s competitive job market, earning a degree is just the beginning. To truly stand out, college students and freshers must constantly upskill, stay updated with industry trends, and gain…
Top Computer Science Jobs for Freshers in India
The rapid evolution of technology has created immense opportunities for fresh computer science graduates. With the IT sector expanding globally, India is one of the top countries offering lucrative and…
How to Answer – ‘What Are Your Strengths and Weaknesses?’
Landing your first job is a thrilling yet daunting experience. You’ve meticulously crafted your resume, researched the company, and prepped for potential questions. But there’s one question that throws even…